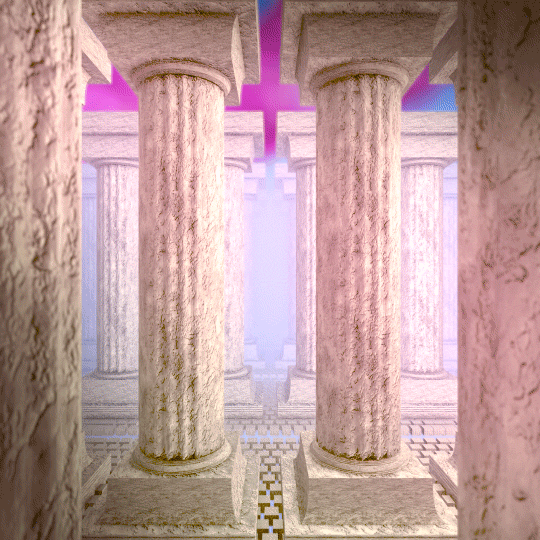
Previously in this series
There's a word for content strategists who don't use data: Writers.
Writing is fine work, no shade intended. But for most of us in the contemporary content biz, developing content pillars without data is a best guess, and usually a wrong one at that.
Incorporating data is crucial for building a 2022-ready content strategy, and audience behavioral patterns and algorithmic insights are widely available to content strategists in the form of web analytics, trends tools, and free survey software. If you have the budget, additional data can be mined through subscription SaaS tools that scrape the web or distill social chatter.
With readily available audience and algorithmic data, content strategists can
- understand what algorithms see
- incorporate what audiences want
- mix in business and brand goals
- organize that information into buckets or themes that guide content creation, aka content pillars.
Content pillars make incorporating audience data manageable for long-term organic growth. Many publishers can't deeply keyword research every single article they publish or create a TikTok explainer for every fad, and that's ok! Incorporating data into strategic pillars that guide content creation for 1-2 years is enough for most publishers or content marketers. It certainly makes for a more cohesive, less frantic, high performing long-term content strategy.
Ideally, a holistic 2-year content pillar approach incorporates quantitative and qualitative data from multiple sources. Depending on resources, these pillars can be developed over a period of three days or several months.
To build pillars, use current, specific data. Personas or surveys from 3 years ago don't cut it to describe audience needs in "typical" years, let alone the massive behavioral and cultural shifts we've seen since March 2020.
Algorithmic data represents audiences at large
For effective content pillars that show up on algorithm-driven platforms, brands and publishers need to understand the human inputs that produce algorithmic results.
Organic content recommendation algorithms in search and social mine mass data from across the web and within their platforms, identifying the semantic patterns attached to a topic that spark audience engagement. In the early 2010s, these processing algorithms could only understand text. But in recent years major platform recommender algorithms have evolved to include image, video and voice inputs.
Comprehending the same volume of data as Google, Facebook or TikTok is out of reach for us mere humans. Luckily we're equipped with actual brains that understand complex realities without translating all inputs to math first. If we sift through even some of the same audience data the algorithms process, we can draw similar conclusions about our audience to inform content pillars.
What data can help us understand how algorithms will process our content pillars? With all things analytics and reporting, it's easier to consider the questions data from algorithmic sources can help publishers answer.
Harnessing algorithmic data to inform content pillars
Start with a list of target subjects that you're considering incorporating into content pillars. Then answer:
- What words do people use to search for the topics you're planning on covering?
- Does topic performance change over time? Does it have seasonality? Is it trending up or down?
- What other topics are related algorithmically to your targets? If you're assessing a cross-channel strategy, how do these related topics differ across platforms?
- Do you currently have authority or algorithmic visibility on any topics, hashtags or search terms?
- Who else creates the most visible content for your target topics? What tropes, language, and formats do they use? Why do you think it's algorithmically successful? (Pay attention to what a computer would record: patterns, what's like/unlike other sources, what's unique or similar about a topic.)
Where does this data come from? Google shares a good deal about keyword inputs for free, and third-party SaaS scrape both search and social content APIs for common keywords and hashtags. My suggestions to get started:
- Basic: Google Trends. Pinterest Trends. Google Search Console. Manual social listening and searching on target topics.
- Upgrades: Keyword research tools like SEMRush, Ahrefs, Mangools, Clearscope, MarketMuse. Social listening and audience intelligence tools like RivalIQ, RiteTag, Brand24, Crowdtangle, Buzzsumo, SparkToro.
- Fully loaded: Enterprise SEO software: BrightEdge, SearchMetrics, Conductor. Enterprise social listening software: Sprinklr, Mention, BrandWatch, SocialBakers, NetBaseQuid.
Note: I do not recommend Google autocomplete question scrapers like Answer the Public for high-level topic research. Autocomplete search data is great for assessing the long-tail, but when developing pillars we want to focus on head terms with more volume.
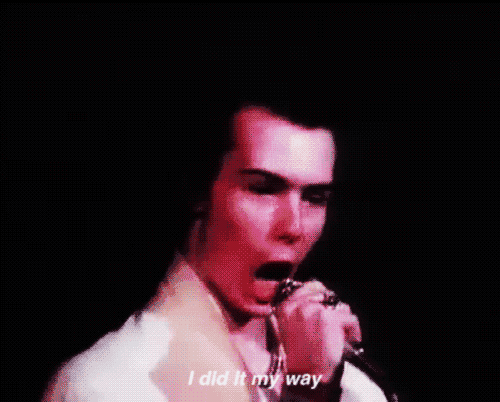
More on entity and topic search authority
Your content is unique, and your audience data should be too
Audience digital behavior regularly defies my assumptions, despite a decade of diving into analytics and trends reports. Even with deep editorial, web and SEO experience, if I were to glance at a website and choose five pages that fostered engagement or produced revenue, I'd maybe get one right without checking analytics first.
Every audience is unique, and content pillars should be developed with your audience's specialized needs and behaviors in mind. Industry benchmarks, whether they originate from Google Analytics or trade organization surveys, just don't cut it for audience input. Even within similar industries, the content and channel mix that works for one brand is often wildly different than what succeeds for another.
Assessing your audience data for content pillar development
When developing content pillars, try to answer these questions with your owned audience data. You can conduct a massive content audit, or you can just look at your top 10 or top 50 pieces of content and draw your own conclusions. You don't need to be a trained analyst as long as you can navigate the basics of an analytics platform and read a chart.
To inform pillars, answer the following:
- What content do users spend the most time with? How do those differ from the content that attracts the most traffic?
- What subjects attract traffic or engagement consistently, regardless of time of year or virality?
- What content does your target audience tell you they want to see, either through surveys or direct response?
- What content attracts new followers or visitors versus what keeps returning visitors coming back?
- What content produces business value, like subscriptions, leads or ad revenue?
Reliable sources for owned data analysis:
- Basic: Web, email and social analytics tools are essential and free.
- Upgrades: Surveys or customer service/chat logs.
- Fully loaded: Focus groups or user interviews.
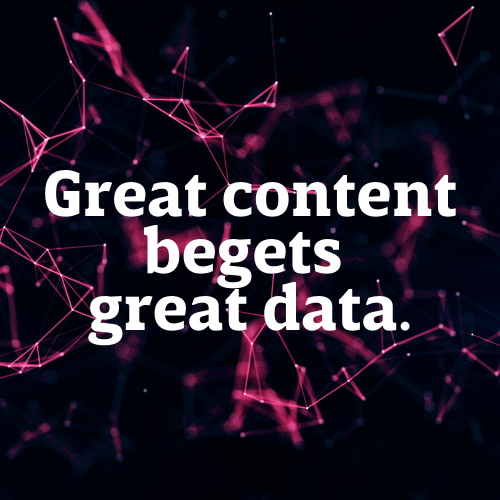
More information on methods for gathering owned data
Matching your content goals with brand vision and business needs
Armed with the data of algorithmic and audience research, you're ready to get rolling on your content pillars... but first, spend some time with your business goals.
Be clear about your brand mission, vision and/or values statements. I can't help you with defining your brand (Google can!), but I can tell you that writing a list of brand values is extremely helpful when defining pillars.
Even if you're just a solo content creator, take some time to consider what you stand for and how you want to represent yourself through content. Don't decide on pillars just because they're popular; choose the terms and topics that align best with your brand vision. To keep the whole thing sustainable, also make a note of the topics that bring you joy alongside the ones that make you money.
You don't need an MBA to determine what your business goals are, but if you want to keep the lights on, you have to know how you're paying the bills. The content pillars you choose should ultimately support these business goals along with your audience needs.
Business questions to answer before developing content pillars
- How does your content generate value (i.e., growing an audience of subscribers, attracting traffic for ads, driving quality leads, recruiting staff)?
- What topics align with your brand values? How can you better represent your values in the topics you've chosen?
- What topics or points of view differentiate you from competitors?
- Resource-wise, what are you capable of covering sustainably?
- Where do you aspire to be in the next 1-2 years?
Sources for your business data:
- Basic: Your preferred note-taking app (see below). Conversations with your colleagues. Helps if you have notes from the course of the past year, but a blank page works too.
- Upgrade: Business plan, mission/vision/values statements and revenue pipeline.
- Fully loaded: Quarterly forecasts of content performance goals for the next two years.
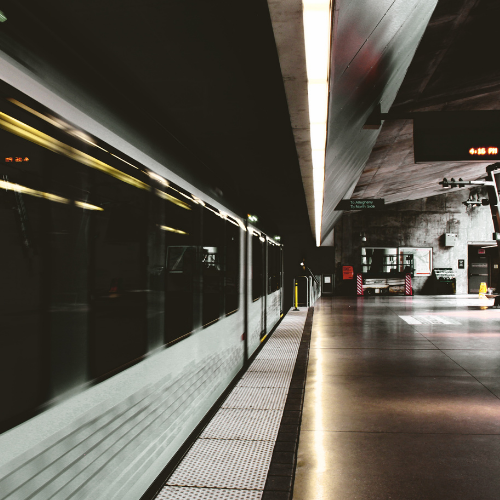
Content business model breakdowns and the software that supports them
Triangulating your data to create content pillars
Now that you have gathered the pieces, the content pillar building process looks something like this:
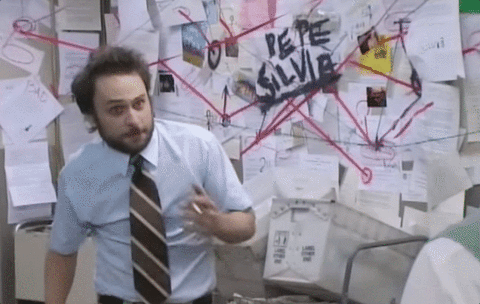
After you've spent a while answering the questions above, you should start to see themes and connections among topics that perform, audience needs and desires, algorithmic considerations, and what makes sense for your business.
Remember that pillars are the topics and ideas for which you want to be known as a brand. For your sanity, consider that not every pillar needs to be deeply performance-focused; some can simply be aligned with brand, like a pillar that celebrates employee accomplishments or the one that's just pure fun.
When putting it all together, keep the following in mind:
- Selecting pillars that incorporate at least two of the three types of data mentioned above. For example, an Employee Accomplishments pillar hits both brand values and high performance (that stuff is built for LinkedIn!), even if it's not an algorithmic priority.
- Align with teammates, stakeholders and higher-ups on the reasons and rationales for each pillar type. Come prepared with examples and data.
- Pillars are meant to be future-proof, at least in the 1-2 year short term. That said, if a pillar's not hitting performance targets six months in, you may want to have an alternate waiting in the wings.
In the new year: measuring pillar performance across channels, for paid/founding members only.
Hand-picked related content
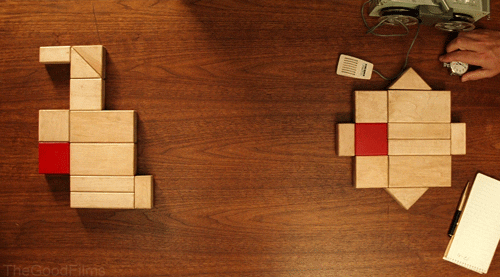
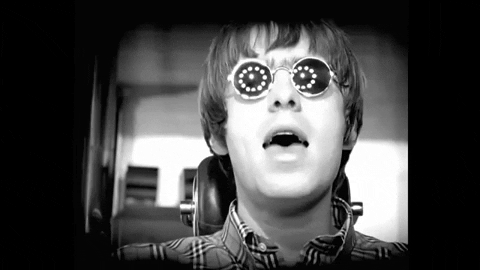